Exploring Quantitative Investing Strategies and Insights
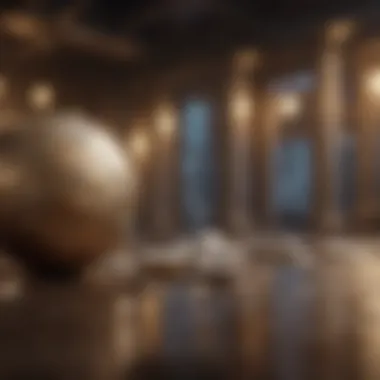
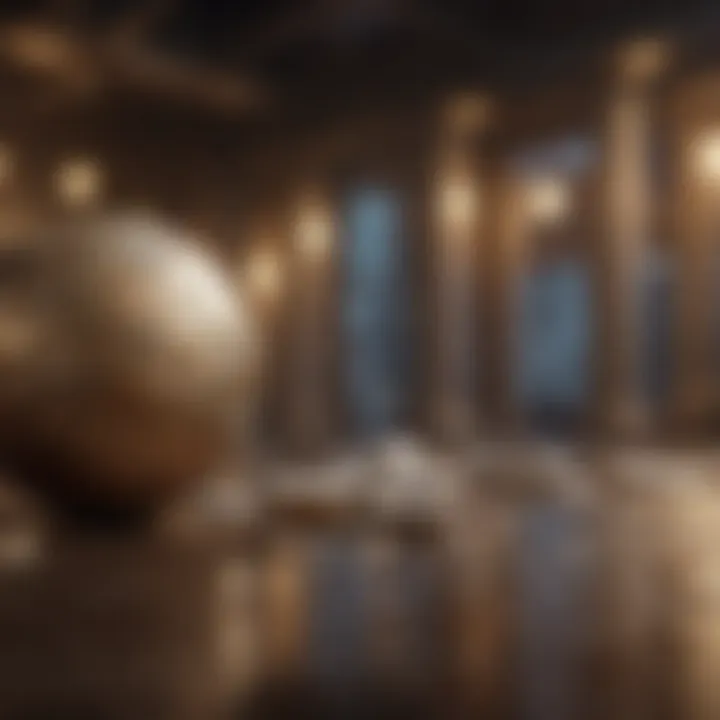
Intro
Quantitative investing stands as a compelling approach amidst the sprawling landscapes of modern financial markets. Unlike traditional investing which often leans on intuition or qualitative analysis, quantitative investing relies heavily on mathematical models and statistical analysis to inform investment decisions. With the explosion of data in recent years, fueled by advancements in technology and increasing computational power, the quantitative investing sphere has gained remarkable momentum.
This investing strategy is driven by complex algorithms and comprehensive datasets that attempt to predict market movements. The need for accuracy and speed makes quantitative methods indispensable for some investors, particularly those keen on maximizing their returns while minimizing risks. In this article, we will explore the various components that come together to form the backbone of quantitative investing, as well as the potential impacts on individual investors keen for smart capital allocation.
As the world continues changing and markets evolve, understanding the nuances of this investing method becomes not just beneficial but essential for financial enthusiasts and individual investors alike. With the pace at which technology is advancing, the connection between data and successful investment strategies is tighter than ever.
By unpacking the fundamentals of quantitative investing, we aim to prepare readers not only to grasp the concept but also to apply it effectively in their own journey towards financial growth.
Prologue to Quantitative Investing
Understanding quantitative investing is pivotal in todayâs financial landscape. It represents a way of making investment decisions based on numerical data and mathematical models rather than gut feelings. In essence, it taps into the power of data analytics to drive investment choices. This method is not just a modern fad; itâs become an indispensable aspect of trading strategies for professional investors.
Defining Quantitative Investing
Quantitative investing can be boiled down to one simple idea: using quantitative analysis to guide investment decisions. This approach involves analyzing numerical data to identify trends, assess risks, and make predictions about future price movements. Itâs about crunching numbers to derive insights that are often overlooked in traditional investment strategies. Investors leveraging quantitative methods use statistical processes to scrutinize historical data, aiming to spot patterns that can suggest where markets might head next.
This style of investing doesnât stop at simple metrics; it encompasses complex algorithms and advanced models designed to evaluate countless variables simultaneously. Investors often rely on software tools that automate the analysis, which speeds up the decision-making process. Just imagine having the ability to sift through terabytes of data in mere secondsâthis is the kind of magic quantitative investing brings to the table.
Historical Context and Evolution
The roots of quantitative investing date back several decades, gaining traction especially in the late 20th century. Early methods primarily revolved around technical analysis, where traders examined historical price and volume data. However, as computing technology advanced, so did methodologies. By the 1980s and 1990s, with the rise of sophisticated statistical analysis tools, investment firms began drafting more sophisticated quantitative models.
Quantitative investing evolved significantly during this period. Pioneers like James Simons with Renaissance Technologies showed the potential for profit when data crunching and programming amalgamate. The systematic approach favored in quantitative strategies gradually infiltrated the broader investment landscape.
Today, we find quantitative strategies firmly entrenched in Wall Street and beyond, accompanied by new tools that evidence data-driven insights like never before. This historical trajectory highlights not only the importance of technology but also the relentless evolution of investment strategies to adapt to changing market conditions.
The Core Principles of Quantitative Investment Strategies
Quantitative investing leans heavily on data and statistical methods, making the core principles essential for success in this complex landscape. These principles not only provide a framework for making informed investment choices but also help in developing a disciplined approach to trading and asset management. Understanding these foundational concepts can sharpen an investor's analytical abilities and enhance decision-making processes.
Data-Driven Decision Making
At the heart of quantitative investing lies data-driven decision making. This approach pivots away from gut feelings and subjective analysis; instead, it relies on historical data, financial metrics, and quantitative models to inform strategies. By harnessing vast volumes of data, investors can identify patterns and correlations that might be invisible to the naked eye.
For instance, imagine an investor analyzing stock price movements over the past decade. By employing statistical techniques, they can spot trends that denote potential future performance based on historical behavior.
Some key elements that underscore the significance of data-driven decisions include:
- Informed Choices: Analytical insights derived from data help in making sound investment choices, reducing reliance on emotional responses.
- Scalability: Techniques that utilize data can be scaled to process vast datasets efficiently, driving faster decision-making.
- Verification: Data allows for backtesting strategies under various market conditions, thus validating their effectiveness before real-world application.
Statistical Models and Algorithms
Statistical models and algorithms are the very backbone of quantitative strategies. These computational tools transform raw data into intelligible insights and actionable strategies. A common practice involves employing models like linear regression, time-series analysis, and machine learning algorithms to forecast price movements and eliminate assumptions based on randomness.
For example, linear regression could help an investor ascertain how much the price of a particular asset responds to changes in economic indicators. Algorithms come into play not just in analysis but also in trade execution; high-frequency traders often employ sophisticated algorithms to take advantage of fleeting trading opportunities when market conditions align favorably.
Consider the following benefits:
- Predictive Power: Statistical models allow for predicting future outcomes based on past behavior, ideally enhancing investment performance.
- Minimization of Bias: Algorithms eliminate human bias by relying strictly on data and established criteria.
- Dynamic Adjustments: Algorithms can adapt to changing market conditions in real-time, offering a strategic edge.
Risk Management Techniques
Effective risk management is critical in quantitative investing. Identifying and mitigating risk factors can protect portfolios from unforeseen market shifts. Quantitative strategies incorporate various risk management techniques to safeguard investments without compromising potential returns.
Some common methodologies include:
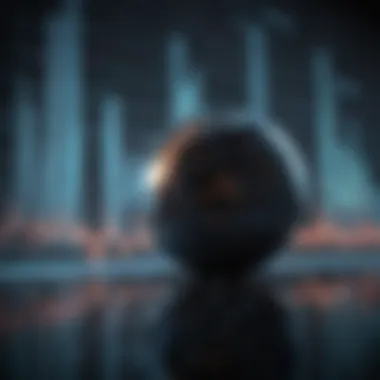
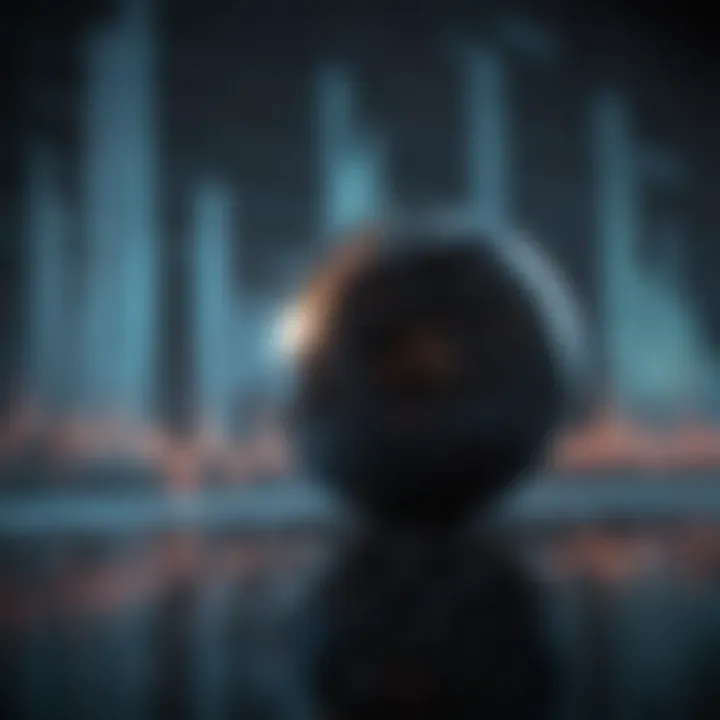
- Value at Risk (VaR): This statistical technique estimates the potential loss in value of a portfolio over a defined period for a given confidence interval.
- Diversification: By spreading investments across a range of assets, investors can mitigate risks. Quantitative models can pinpoint optimal diversification levels.
- Stop-Loss Orders: These predefined boundaries help limit potential losses by triggering a sale at certain prices, effectively creating an automated safety net.
Keeping an eye on these risk management techniques can bolster an investor's chances of sustaining long-term profitability in the often turbulent waters of financial markets.
"Investing without research is like playing a game without knowing the rules."
Types of Quantitative Investing Strategies
Understanding the various types of quantitative investing strategies is paramount for any investor seeking to navigate the complexities of todayâs financial markets. These strategies are rooted in systematic data analysis and mathematical models, which offer structured frameworks for making investment decisions. By categorizing the strategies, investors can better comprehend their unique characteristics, advantages, and applicable scenarios. This segment delves into four primary strategies: trend following, mean reversion, statistical arbitrage, and machine learning applications, each with its own set of methodologies and implications for decision-making.
Trend Following Strategies
Trend following is a powerful strategy that aims to capitalize on the momentum of price movements in the markets. The essence of this approach is simple; if a market is trending upward, the strategy suggests buying, and if itâs trending downward, the recommendation is to sell or short the asset. This methodology relies on historical price data to determine existing trends, often using moving averages or momentum indicators to signal entry and exit points.
One of the critical advantages of trend following is its ability to identify and exploit long-term market movements rather than short-lived fluctuations. This strategy typically aligns with the adage that "the trend is your friend." Notably, trend following can generate substantial returns during strong market moves.
However, investors should be mindful of potential pitfalls, including whipsaw effects during sideways market conditions where price may oscillate without a defined trend. Hence, while this strategy has proven successful over time, it demands a solid understanding of risk management principles to navigate its challenges effectively.
Mean Reversion Strategies
Mean reversion strategies are built on the premise that asset prices will revert to their historical average over time. This approach often entails identifying overbought or oversold conditions using statistical measures. Investors employing a mean reversion strategy typically look for assets that exhibit price anomalies, which might not align with their intrinsic value.
The strength of this strategy lies in its assumption that extremes in the market cannot hold indefinitely. For instance, if a stock is trading significantly below its historical average, a mean reversion approach suggests a buying opportunity, anticipating that the price will eventually bounce back. Conversely, if an asset is overvalued, selling might be the better option.
However, investors must exercise caution, as markets can remain irrational longer than one might expect. Itâs vital to leverage robust statistical analysis and not to be too quick in jumping back into market norms, especially during increased volatility.
Statistical Arbitrage
Statistical arbitrage, or stat arb as itâs often called, is a sophisticated strategy involving the construction of diversified portfolios using statistical methods. This framework looks for discrepancies in relative pricing among correlated assets. For instance, if two stocks are historically correlated but begin to diverge, a stat arb investor might buy the undervalued asset while short-selling the overvalued one in anticipation of a correction.
This strategy allows for capturing small price inefficiencies before they correct themselves, ideally resulting in profits regardless of overall market direction. Nonetheless, statistical arbitrage strategies require significant computational resources and real-time data analysis, making them more suited for institutional investors with advanced technology capabilities.
Understanding correlation dynamics and ensuring a well-balanced portfolio are critical to minimizing risks associated with this strategy. Like a well-tuned machine, precise execution makes all the difference in maximizing profits.
Machine Learning Applications
The rise of machine learning has introduced exciting prospects for quantitative investing strategies. By employing algorithms capable of learning from vast data sets, investors can identify complex patterns that traditional models might overlook. Machine learning applications can automate trading decisions, enhance prediction accuracy, and even adapt dynamically to changing market conditions.
These algorithms analyze historical data, news sentiment, and multiple other variables to inform investment choices continually. Notably, machine learning has empowered investors to explore high-dimensional data and vast numbers of variables simultaneously, which can uncover hidden relationships needed for informed decision-making.
However, as with any advanced approach, machine learning comes with its own challenges, principally concerning model risk. Overfitting, or creating models too tailored to historical data, can lead to poor performance in live trading scenarios. Therefore, while the potential is vast, itâs critical to maintain a grounded perspective and robust validation processes to guard against these pitfalls.
"Data doesnât tell you what to do; itâs the question you ask it that matters."
In summary, these types of quantitative investing strategies serve distinct purposes and come with unique benefits and challenges. Investors should choose a strategy aligned with their risk profiles, investment horizons, and market outlook to optimize their effectiveness in the quantitative investing realm.
The Role of Technology in Quantitative Investing
In todayâs rapidly evolving financial landscape, technology serves as the backbone of quantitative investing. The intersection of finance and technology has profoundly influenced how investors analyze data, execute trades, and manage risk. This section highlights pivotal aspects of technology's role in the realm of quantitative investment strategies, shedding light on its benefits and considerations.
Big Data and Financial Analytics
Big Data is more than just a buzzword; itâs the lifeblood of quantitative investing. The vast volumes of data generated daily from multiple sourcesâranging from market transactions to social media feedsâare imperative for effective analysis. Investors who harness this wealth of information can identify trends, make predictions, and strengthen their investment decisions.
Data analytics tools enable investors to glean actionable insights, facilitating a level of scrutiny that manual methods simply cannot attain. As such, machine learning algorithms come into play, allowing for the processing and interpretation of data at an unprecedented scale. To put it plainly, more data can yield richer insights. However, quantity must be matched with quality. Poor data can lead to misguided conclusions and, ultimately, losses.
"The biggest challenge in Big Data is not the data itself, but making sense of it to derive value."
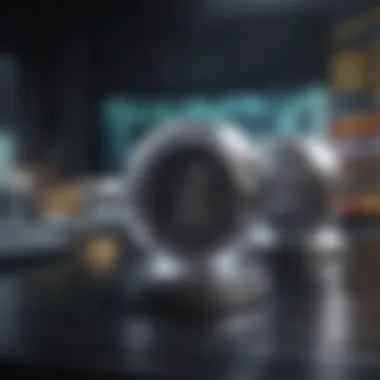
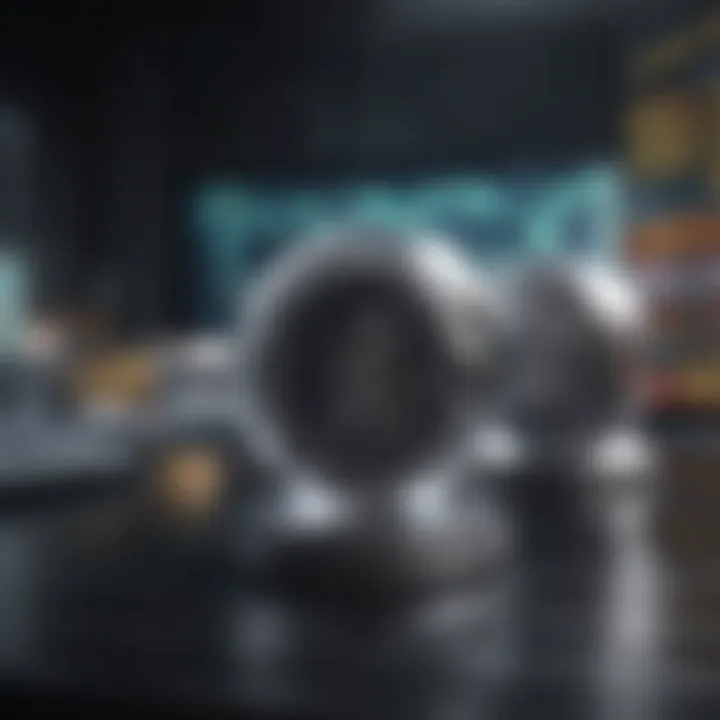
Algorithmic Trading Platforms
Algorithmic trading platforms are at the forefront of automating investment strategies. By leveraging programming to execute trades based on pre-defined criteria, these platforms enhance efficiency and speed. For instance, a trader can set rules based on price movement or market sentiment, allowing trades to occur in milliseconds, a feat that is nearly impossible for human traders to match.
These platforms also allow for greater scalability beyond what individual investors can do. Algorithms can process vast amounts of information, assess risk, and consider market conditions in real-time. However, the reliance on algorithms raises questions about their adaptability to sudden market changes. A flash crash, for example, can catch even the most sophisticated algorithms off guard.
Backtesting and Simulation Techniques
Backtesting is a crucial component of quantitative investing. By simulating trading strategies against historical data, investors can evaluate potential performance before risking any capital. This method not only uncovers the viability of a strategy but also allows for adjustments based on past results. Itâs like having a time machine that lets you test your investment ideas in hindsight.
However, itâs essential to approach backtesting with caution. The risk of overfitting is significant; that is, a strategy might perform well on historical data but fail in real-world conditions due to unforeseen variables. Therefore, implementing robust simulation techniques that account for a variety of market scenarios is vital to ensuring the reliability of quantitative strategies.
In summary, the role of technology in quantitative investing is multifaceted and indispensable. By harnessing the power of Big Data, automated trading platforms, and rigorous backtesting methods, investors position themselves for greater success in an increasingly complex market.
Advantages of Quantitative Investing
Quantitative investing comes with a handful of distinct advantages that stand out in the bustling world of finance. By applying statistical models and advanced algorithms, this approach offers solid benefits that individual investors and fund managers can capitalize on. Understanding these advantages is crucial as they can significantly enhance investment decisions, leading to more informed and effective strategies.
Objective and Systematic Approach
One of the most compelling features of quantitative investing is its objective and systematic nature. Unlike traditional investment strategies, which may rely heavily on intuition or subjective judgment, quantitative investing leans on data. Itâs as if the strategy has a mind of its own, crunching numbers and statistics to establish a clear pathway for decision-making.
In this context, investors can look at historical data and specific indicators to inform their choices. For instance, rather than guessing the outcome of a market trend, quantitative investors analyze trends using historical price movements and market fluctuations. This results in an approach that minimizes emotional biases, such as greed and fear, which often lead to poor decisions in volatile markets.
"In a world flooded with information, itâs the systematic approach that cuts through the noise, allowing investors to navigate the complexities of financial markets with clarity."
Enhanced Risk Assessment
Another notable advantage is the enhanced risk assessment that quantitative methods provide. Through rigorous analysis, quantitative investing helps identify potential risks in various securities or strategies. Models can simulate different market conditions, providing insight into how portfolios might behave under stress or turbulent waters. This is essential for determining risk-adjusted returnsâessentially evaluating how much risk an investor is willing to take for the potential of earning returns.
By utilizing techniques like Value at Risk (VaR) and Monte Carlo simulations, investors can gain a clearer picture of the risk landscape. For example, if an investor uses these methods to evaluate a tech stock, they may find that the potential for loss at a certain confidence interval is higher than previously thought. Knowing this beforehand empowers investors to make adjustments, such as diversifying their holdings or temporarily reallocating funds, which could cushion their portfolio against sudden downturns.
Efficient Market Analysis
Lastly, the efficiency of market analysis shines brightly in quantitative investing. With technology advancing rapidly, the amount of data available today is staggering. Quantitative strategies harness this data effectively, analyzing trends and patterns that are sometimes invisible to the naked eye. This allows investors to forecast market movements more accurately and spot opportunities before they become obvious to the broader market.
Quantitative investors often look into variables that are not only financial but may also combine behavioral data, such as social media sentiment or economic indicators. For instance, if sudden negative sentiment arises regarding a particular stock, a quantitative strategy might detect this trend through vast datasets that help anticipate a price drop. By leveraging such insights, investors can position themselves more advantageously, optimizing their portfolios based on solid evidence rather than mere speculation.
Challenges in Quantitative Investing
In the realm of quantitative investing, it's crucial to recognize the hurdles that come along with this analytical approach. While quantitative strategies hold the promise of systematic investing, they are not without their challenges. Navigating these obstacles can often determine whether an investment strategy thrives or falters.
The importance of addressing these challenges lies in their direct impact on the effectiveness of quantitative models. Investors need to ponder how data quality, model risks, and market adaptability factor into their strategies. When investing is based solely on models, overlooking these issues can lead to significant losses. Thus, this section dives into the intricacies of these challenges, so investors can fine-tune their approaches and maximize their outcomes.
Data Quality and Availability Issues
In quantitative investing, data is the backbone. If the data isn't up to parâwhether in terms of accuracy, completeness, or timelinessâthe foundation of any model is shaky at best. Data quality issues can arise from various sources, including human errors in data entry and misaligned datasets that can lead to false conclusions. For instance, investment firms often deal with big datasets derived from multiple sources, and integrating them can be like fitting a square peg in a round holeâit just doesnât work.
Moreover, data availability can present another layer of difficulty. Certain financial metrics may simply not be accessible due to proprietary concerns or outdated systems. When certain data points are missing, the investor faces a significant challenge: either to proceed with an incomplete model or to invest time and resources into resolving the gaps.
To mitigate these issues, quantitative investors must rigorously vet their data sources, ensuring reliability. As a saying goes, âgarbage in, garbage out.â High-quality data is essential for producing meaningful insights, and thus, being selective about data acquisition is a fundamental practice.
Model Overfitting Risks
Model overfitting is basically a system that tries to weave a fine fabric but ends up with a patchy result. When a model is too complex, it tends to tailor itself closely to historical data, capturing noise rather than the underlying trends. This leads to an illusion of accuracy, convincing investors they've found the golden ticket, only to end up disappointed when they face real-world conditions.
It's all too easy for investors to write off these misfires, often claiming that the model was âcloseâ enough. However, this could result in poor forecasting and regrettable investment choices. Overfitting is not merely a technical flaw; itâs a fundamentally misleading statistic that underscores the importance of balancing model complexity and generalization. Keeping models simple, yet effective, is key. Investors must constantly validate their models against out-of-sample data to guard against overfitting and avoid overly complicated algorithms that donât serve the actual market.
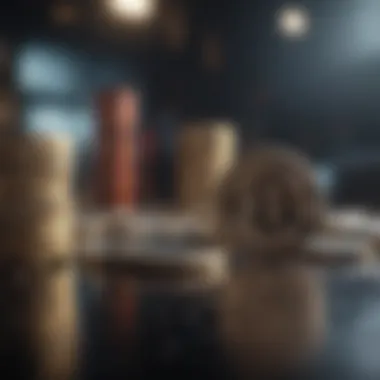
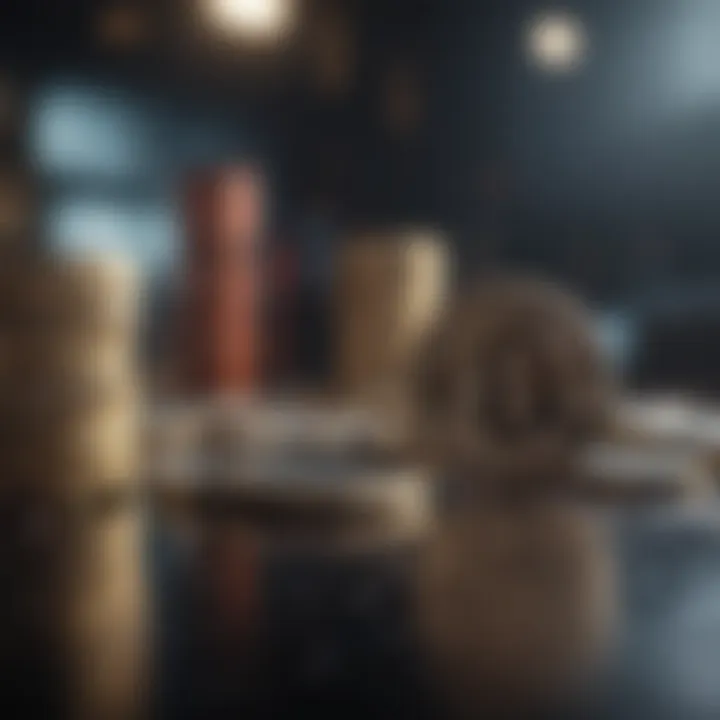
"Overfitting a model is akin to making a suit tailored only to one specific body type; itâs ill-suited for broader use.â
Market Changes and Adaptability
Market conditions are as fluid as water in a stream. New regulations, economic shifts, or unforeseen events can disrupt even the most well-laid quantitative strategies. What worked yesterday may not work today, so a static approach is often a recipe for disaster. This lack of adaptability creates a precarious situation where quantitative investors are forced to respond, often scrambling to reassess methodologies mid-stream.
Investors must foster a sense of flexibility in their quantitative strategies. Rigid adherence to models without conducting regular recalibrations can lead to missed opportunities or, worse, net losses. Engaging in periodic model assessments and ensuring that algorithms can withstand stress tests under varied market conditions is paramount.
In summary, while quantitative investing offers numerous benefits, itâs also laden with its share of challenges. Investors who recognize and confront these issuesâdata quality, model overfitting, and market adaptabilityâcan improve their investment outcomes. Essentially, itâs about treading carefully in a landscape filled with potential pitfalls.
Ethical Considerations in Quantitative Investing
As quantitative investing continues to gain traction in the financial world, discussing its ethical implications becomes crucial. While the advantages of using data-driven approaches cannot be ignored, practitioners must reflect on the broader consequences of their strategies. Ethical considerations ensure that investors remain accountable for their decisions and the impact those decisions have on the market and society at large. Specifically, two vital components in this realm are transparency and fairness, alongside examining the influence of algorithmic trading on overall market dynamics.
Transparency and Fairness
When discussing transparency, the spotlight often shines on how quantitative strategies utilize vast amounts of data. Investors and firms wielding such strategies must maintain clarity regarding how data is sourced and used. For instance, suddenly utilizing private or non-public information could flag serious ethical concerns, even if the strategy itself appears mathematically sound.
Transparent practices boost credibility and trust, not only among clients but also within the broader community. When the methodology behind trading decisions remains undisclosed, potential conflicts of interest emerge. Investors should feel secure that the algorithms driving their portfolios are not influenced by questionable motives.
Amidst the rise of algorithmic trading, fairness stands as another pillar of ethical consideration. The financial market thrives on liquidity and efficiency, but practices such as "high-frequency trading" can disrupt this equilibrium. If certain players gain an edge purely due to technology, smaller investors might find themselves at a disadvantage. This discrepancy raises concerns over whether the market operates on a level playing field.
To cultivate a fair environment in quantitative investing, firms should engage in practices like:
- Regular audits of algorithms to ensure compliance with ethical standards.
- Public disclosures regarding potential biases in data and how they might affect outcomes.
- Encouraging feedback from regulators and the investing public to further unveil transparency issues.
Impact of Algorithmic Trading on Markets
Algorithmic trading has revolutionized how trades are executed, replacing human intuition with complex algorithms. While this may enhance efficiency, several ethical concerns bubble beneath the surface. For one, the speed at which algorithms operate can sometimes be to the detriment of market stability.
Investors might ask: Are these algorithms merely enhancing competition, or are they exacerbating volatility? The 2010 Flash Crash serves as a stark reminder of how algorithmic trading can magnify disruptions. In a matter of minutes, the Dow Jones Industrial Average plummeted, wiping out hundreds of billions in value.
Furthermore, market manipulation via algorithms is another dark alley that investors must be wary of. Techniques like quote stuffingâwhere traders swiftly submit and cancel large ordersâcan create confusion and mislead other market participants, impacting price discovery.
"The ethical implications of algorithmic trading extend beyond mere performance; they touch the very fabric of market integrity."
To address the challenges posed by algorithmic trading, tangible steps can be taken:
- Encourage regulatory bodies to implement stringent regulations that govern algorithmic trading practices.
- Foster an open dialogue about market integrity, bringing together industry leaders, regulators, and technologists.
- Develop tools and frameworks aimed at enhancing both transparency and accountability around algorithmic decisions.
In summary, ethical considerations in quantitative investing demand ongoing dialogue and diligence within the investment community. As the landscape evolves, the frameworks set in place today will shape the future of investing and the market's integrity.
Future Directions in Quantitative Investing
As we peer into the looking glass of quantitative investing, it's clear that the landscape is shifting rapidly. The convergence of technology, advanced analytics, and evolving market behaviors is not just a trend but rather a wave of transformation. This section delves into the future directions that quantitative investing is poised to take, emphasizing the innovations that could redefine the investment practice.
Advancements in Artificial Intelligence
Artificial intelligence is akin to a powerful engine driving the next phase of quantitative investing. The algorithms that perform analyses are becoming more sophisticated, allowing for better prediction and decision-making. For instance, machine learning techniques help in identifying patterns in vast datasets much faster and more accurately than human analysts ever could.
A prime example of this is the use of neural networks, which can model complex relationships within data. These networks learn from past data and improve their predictions over time. Moving forward, we are likely to see even more integration of AI in portfolio management, where systems can operate with minimal human intervention, adjusting strategies in real-time according to market shifts.
Integration of Behavioral Finance
Despite the emphasis on numbers and algorithms, human behavior remains a critical factor in the markets. Integrating behavioral finance into quantitative models represents a promising frontier. This branch of finance examines psychological influences on investor decisions, which can skew market performance.
By merging this field with quantitative investing, we can better understand anomalies, like why stocks sometimes behave irrationally. For example, during periods of panic, stock prices might fall contrary to fundamental performance indicators. A quantitative model that incorporates behavioral insights can potentially mitigate this by predicting such behavior before it manifests. Knowing when sentiment is likely to sway can be a game changer for investors.
Collaboration of Human Intuition with Algorithms
While algorithms can process vast amounts of data, they often lack the nuanced understanding that a seasoned investor might possess. The future of quantitative investing could lie in a hybrid model that marries human intuition with algorithmic efficiency.
This collaboration allows human analysts to guide algorithms, ensuring they remain aligned with market realities. Furthermore, human insight can refine models, providing context that raw data alone cannot supply. In this way, the best outcomes emerge from a partnership where humans and machines learn from one another, creating a feedback loop of continuous improvement.
"The future of investing isn't just about algorithms; it's about finding the harmony between human judgment and automated precision."