Understanding Symmetric Distribution in Investment Analysis
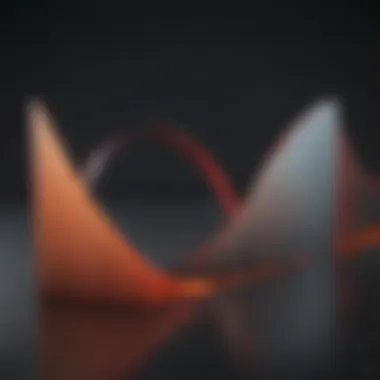
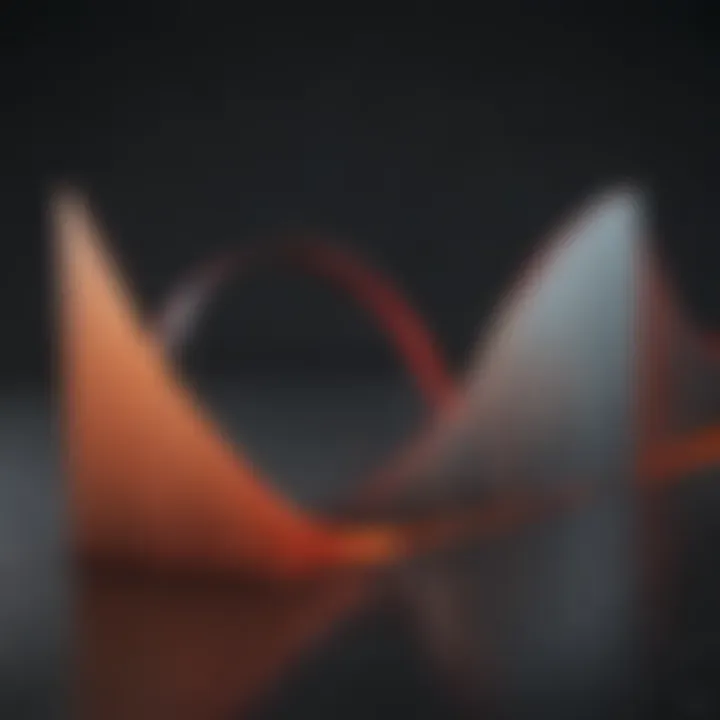
Intro
When one delves into the world of investments, navigating through various statistical tools can be a daunting task. Among these, symmetric distribution stands out for its applicability and significance in financial analysis. At its core, symmetric distribution helps investors understand how returns are expected to behave. Knowing this is crucial for making informed decisions, especially when it comes to risk assessment and capital allocation.
A symmetric distribution isn’t just an abstract concept; it reflects how collective behavior could lead to patterns within market returns. For instance, if we think about a bell curve—the most common representation—it's easy to envision how outcomes can cluster around a central point, with fewer occurrences as we move away from the mean. However, it's not merely about aesthetics. Understanding the implications of this kind of distribution is pivotal for anyone serious about investment.
Investors often look for patterns and trends, but those can be misleading without a robust grasp of distribution. This article aims to demystify symmetric distribution, providing insight on its characteristics, relevance in risk management, and practical applications within the investment framework. With real-world examples and detailed discussions, we hope to elevate your analytical thinking in decision-making processes.
Understanding Symmetric Distribution
When analyzing investments, grasping the notion of symmetric distribution is crucial for any investor keen on making informed decisions. This concept is not just an esoteric statistical theory; it has tangible applications in understanding market behaviors. Symmetric distribution enables analysts to make assessments regarding risk and return that are key to portfolio management. If one can visualize data that behaves in a symmetric manner, such as price fluctuations or returns on an asset, then the paths for predictive modeling and risk management become clearer.
Definition and Characteristics
Symmetric distribution refers to a type of probability distribution in which the left and right sides of the distribution's graph are mirror images. This characteristic signifies that most values cluster around a central point, usually the mean, median, and mode, which are all positioned in the middle of the distribution. A classic feature of symmetric distributions is that when one plots a graph of the data, it will look the same on both sides of a vertical line through the mean. This balance is what makes it a foundational element in statistical analysis related to investments.
For investors, understanding the characteristics of symmetric distribution helps in better estimating the likelihood of various investment outcomes under normal market conditions. It instills greater confidence in predictive models used to forecast future performance.
Types of Symmetric Distributions
The two most commonly discussed types of symmetric distributions in investment analysis are the Normal Distribution and Uniform Distribution. Each presents unique attributes that make them beneficial in particular contexts.
Normal Distribution
The Normal Distribution is perhaps the most recognized symmetric distribution in finance. It is characterized by its bell-shaped curve, where most of the data points cluster near the mean. This shape signifies that extreme values on either end (i.e., huge gains or losses) are less likely to occur. For investors, deploying normal distribution in quantitative models offers a clear view of expected returns and the probability of potential risks.
One of the appealing aspects of the Normal Distribution is its ability to describe real-world phenomena effectively, from stock returns to measurement errors. This makes it a popular choice for many quantitative analysts. A potential downside, however, lies in its assumption of continuous data and the possibility of underestimating the likelihood of outlier events, often referred to as 'tail risks'.
Uniform Distribution
In contrast, the Uniform Distribution portrays a scenario where all outcomes are equally likely. Its graph appears as a flat line, suggesting that every value within a range has the same chance of occurring. Investors use uniform distribution often when modeling situations lacking historical data or when outcomes are relatively unknown. The key characteristic of uniform distribution is its simplicity—it does not assume a bias toward any specific outcome.
However, while this distribution can be useful in certain uncertain scenarios, it may lack the depth of insights that more intricate distributions provide. Investors relying solely on uniform distribution could risk missing patterns or relationships that only become visible with more complex models.
Understanding these distributions equips investors with crucial knowledge in making educated decisions about risks and returns, allowing for a more robust investment strategy.
Mathematics of Symmetry
The mathematics of symmetry is a cornerstone in understanding how symmetric distributions operate. This section delves into the foundational concepts such as mean, median, and mode, as well as the properties of symmetric functions that underpin much of statistical analysis. Understanding these aspects is crucial for investors who rely on robust mathematical principles to guide their decision-making processes.
Key considerations include the relevance of these mathematical tools in interpreting financial data, conducting risk assessments, and optimizing portfolios. Investors often face an array of complex data; thus, a clear grasp of symmetric functions facilitates better comprehension and application of investment strategies.
Mean, Median, and Mode
The trio of mean, median, and mode are fundamental measures of central tendency that provide insight into the distribution of data points. In the context of symmetric distributions, these metrics hold particular significance as they coincide, offering a reliable snapshot of the dataset.
- Mean: This is calculated by summing all values and dividing by the total number of values. In a symmetric distribution, the mean serves as the balancing point of the data. For example, if one were analyzing the stock returns of a particular company over a period, the mean would represent the expected return, which both investors and analysts utilize for forecasting.
- Median: Reflecting the middle value of a dataset when arranged in order, the median is particularly useful when assessing skewed data. Yet in symmetric distributions, it aligns perfectly with the mean and mode, reinforcing the stability of measurement.
- Mode: Representing the most frequently occurring value within a dataset, the mode elucidates common return scenarios investors might encounter. Its presence in symmetric distributions not only highlights trends but also assists in liquidity considerations.
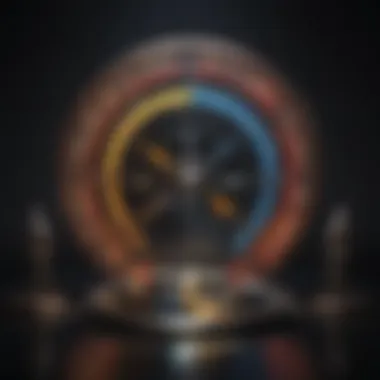
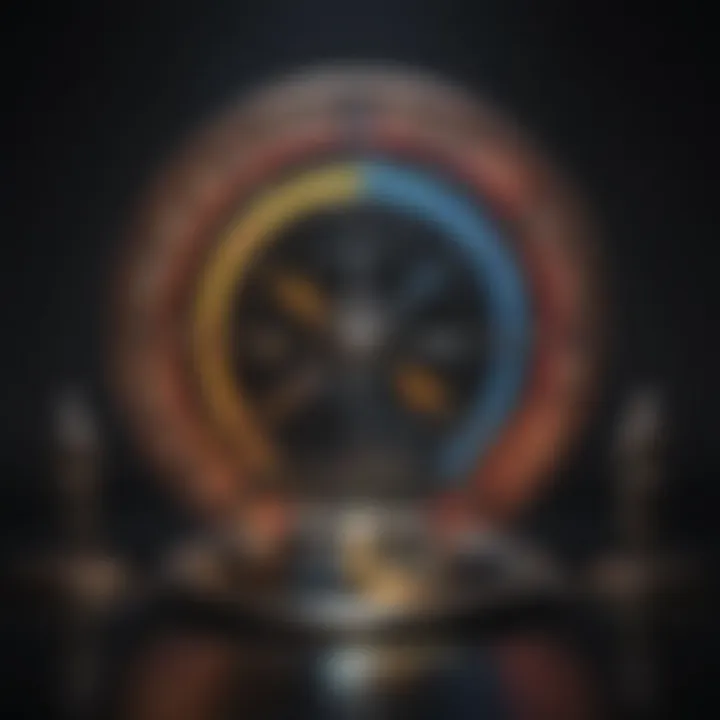
Obtaining a solid understanding of these fundamentals not only enhances one's grasp of symmetric distributions but also aids in the execution of informed investment decisions.
Properties of Symmetric Functions
Even Functions
Even functions represent a vital aspect of symmetric functions, defined by the condition that if ( f(x) = f(-x) ), it indicates that the function reflects perfectly along the vertical axis. This reflection showcases the balanced nature inherent in symmetric distributions. Investors and analysts greatly benefit from this characteristic because it simplifies the modeling of asset returns under symmetric conditions.
- Key Characteristic: The critical aspect of even functions is their predictable behavior, which fosters a dependable basis for financial analysis.
- Popularity in Analysis: Their prevalent usage comes from their ability to model various financial scenarios, making them a popular choice among those engaged in quantitative finance.
- Advantages: Utilizing even functions can streamline calculations, enhance visual representations on charts, and provide a crisp framework for evaluating performance metrics.
Reflection Symmetry
Reflection symmetry describes a scenario where a function or shape appears unchanged when reflected across a specific line. In finance, this tied closely to the construct of symmetric distributions, where the return dataset holds equal probability of falling above or below the mean. Understanding reflection symmetry becomes essential in the realm of investment analysis as it signifies balanced risk and returns.
- Key Characteristic: The defining trait here is the mirroring effect, which assures investors that the expected outcomes hold an equal chance of occurrence.
- Benefit for Investors: When investors comprehend this, they can manage their portfolios more adeptly, knowing there’s a predictable pattern that can drive their strategy.
- Unique Feature: The notable aspect of this property is the intrinsic relationship it maintains with various statistical measures, enriching the analysis of different financial tools and methods while keeping in mind their potential limitations.
The properties of symmetry in mathematical concepts not only deepen the understanding of distributions but also forge pathways for improved financial strategies.
Understanding these mathematical principles allows investors to dissect complex data, reduce uncertainty, and gain a more precise vantage point in their investment pursuits. By weaving together the relevance of mean, median, and mode with the characteristics of symmetric functions, one’s analytical prowess is significantly enhanced. This ultimately leads to more informed and effective investment decisions.
Statistical Implications
Understanding the statistical implications of symmetric distribution is essential for investors and analysts alike. This section will highlight how symmetric distribution influences key concepts in hypothesis testing and the Central Limit Theorem. Both of these elements play critical roles in shaping investment analysis and decision-making by providing a structured approach to interpreting data.
Role in Hypothesis Testing
Hypothesis testing is one of the cornerstones of statistical analysis and carries significant weight in investment decisions. When an investor proposes a hypothesis, such as a stock's return being consistent with a predetermined value, they will typically use symmetric distributions as a framework for evaluating that assertion. These distributions, often representing returns that cluster around a central mean, make it more feasible to apply statistical tests like the t-test or z-test.
The attractiveness of using symmetric distributions here is straightforward. With a symmetric distribution, the probability of extreme values occurring on either side of the mean remains even. This allows for a more robust interpretation when investors seek to determine if a stock's performance deviates in a statistically meaningful way from its historical average.
Some essential points include:
- Balanced Outcomes: Investors often align their expectations in both downturns and upturns of the market, knowing that symmetric distributions imply equal chances for both.
- Standard Error Estimation: The use of symmetric distributions facilitates better estimates of standard errors, which in turn helps refine confidence intervals for predictions.
- Type I and II Errors: Understanding the symmetry in distributions aids in minimizing errors. In hypothesis testing, knowing the underlying distribution can guide the selection of appropriate thresholds for rejecting the null hypothesis.
"Statistical significance can be elusive, but understanding the implications of distribution types can shine a light on our interpretations."
Central Limit Theorem
The Central Limit Theorem (CLT) is fundamental to grasping the power of symmetric distribution in investment analysis. Simply put, the theorem posits that the sum or average of a sufficiently large number of independent, identically distributed random variables will tend to be normally distributed, regardless of the original distribution of the variables. Why does this matter to investors?
When investors aggregate data over time—say, for daily stock returns—the CLT assures them that the average return will approximate a normal distribution as the sample size increases. In practical terms, this means that as the amount of data grows, it becomes easier for investors to apply statistical methods that assume normality. They can thus use tools like value-at-risk (VaR) analysis and portfolio allocation strategies that depend on this assumption.
Some insights related to the CLT in investment scenarios include:
- Risk Assessment: The normal distribution allows investors to quantify risk more effectively, providing insights into potential losses or gains.
- Predictive Power: As sample sizes grow, predictions become increasingly reliable, which supports long-term investment strategies.
- Portfolio Diversification: The CLT underpins the theory of diversification. As assets are added to a portfolio, it’s possible to predict the portfolio's overall return with greater accuracy.
In sum, the intersection of symmetric distribution with hypothesis testing and the Central Limit Theorem forms a robust framework for investors to analyze and interpret financial data rigorously. This understanding not only boosts analytical capabilities but also ensures that investment decisions are guided by sound statistical principles.
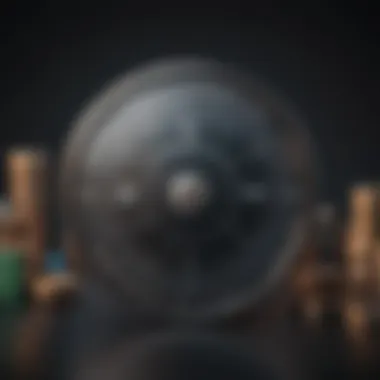
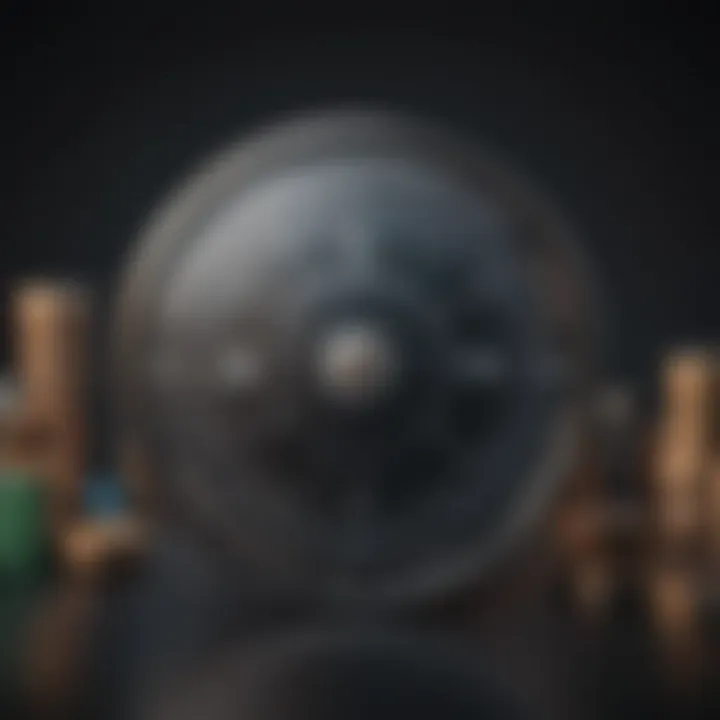
Applications in Investment Analysis
When we talk about symmetric distribution in investment analysis, it’s not just a snooze-fest of theoretical models. It’s about getting a grip on the essential tools that help investors sift through the noise and make sense of financial data. Understanding applications in this realm can really be a game changer for risk management and portfolio decisions.
Risk Assessment and Management
Risk management isn't just about putting out fires; it's about proactively preventing them. In finance, symmetric distributions are particularly useful here. They assist in understanding how assets could behave under the influence of market forces. Because symmetric distributions are, by nature, centered around a mean, estimations of risks can hinge on simpler computations involving standard deviations from that mean.
- Predictable Outcomes: The beauty of symmetric distributions, especially normal distributions, is how predictable outcomes can be. Investors often rely on the bell curve to calculate how much risk they can take. This makes it easier to set up thresholds for losses.
- Decision-Making Framework: Armed with knowledge of symmetric distributions, investors can set more informed limits. Instead of just winging it, they can develop a framework supported by statistical principles. This allows for a more thoughtful allocation of assets.
- Avoiding Panic: For the average investor, the real world can feel like turbulence in a plane. Understanding that returns can vary yet remain largely consistent in pattern can temper emotional decisions during market swings.
Overall, embracing symmetric distributions in risk assessment takes the guesswork out of investing, lending clarity amidst chaos.
Portfolio Optimization
When it comes to portfolio optimization, the math gets intricate, but not in a way that’s mind-boggling. Here’s where symmetric distributions truly shine. They not only facilitate understanding of how different assets will perform over time but also aid in formulating strategies that help in risk-adjusted returns.
Efficient Frontier
At the core of portfolio optimization lies the Efficient Frontier. This concept embodies the optimal allocation of assets in a portfolio so that the expected return is maximized for a given level of risk.
- Visual Appeal: The Efficient Frontier is plotted on a graph where the horizontal axis displays risk and the vertical axis shows expected return. This allows investors to visualize where their portfolios lie in relation to ideal allocations.
- Key Characteristic: One of the appealing aspects? It identifies the portfolios that offer the highest expected return for each level of risk. This helps investors see where they stand and how adjustments could lead to better outcomes.
- Caveat: However, it’s crucial to remember that this concept is grounded in assumptions that may not hold in the real world, especially during times of economic stress. Still, it remains a foundational tool in investment analysis.
Mean-Variance Analysis
Mean-Variance Analysis complements the Efficient Frontier by providing a quantitative measure of risk versus return. In essence, this method breaks down the returns on a portfolio into the expected return and the variance that comes from those returns.
- Emphasis on Balance: This approach focuses on finding the balance between risk and rewards. An investor can decide how much risk they're willing to take, which can greatly shape their investment choices.
- Unique Feature: The beauty of this analysis lies in its ability to help investors construct a portfolio aligned with their risk tolerance. You don’t need to be a math whiz to apply mean-variance analysis; it can show you how adjusting allocations can steer performance.
- Limitation: A downside to keep in mind, however, is that relying solely on historical data can be misleading. Market conditions change, and past performance isn’t always indicative of future results. This underscores why it's important to blend multiple strategies and analyses.
In the tumultuous waters of investing, understanding symmetric distribution and its applications provides crucial insights that help in navigating shifts and trends.
In summary, applying symmetric distribution to investment analysis isn't merely academic. It cultivates a thorough understanding of risk and returns that can steer decision-making in the right direction.
Limitations of Symmetric Distribution
When we examine symmetric distribution in investment analysis, it’s crucial to recognize that, while it provides valuable insights, it also has its share of limitations. Understanding these limitations helps investors and financial analysts avoid pitfalls that could skew their interpretations of data. Here, we’ll dive into two primary limitations: assumptions in financial models and challenges arising from real-world data.
Assumptions in Financial Models
Symmetric distributions often rest on assumptions that can be overly simplistic. For example, many financial models assume that returns on investment are both normally distributed and independent over time. This leads to a few key issues:
- Normality of Returns: The assumption that asset returns are normally distributed implies that extreme events are rare. However, anyone who has kept an eye on the stock market knows this isn't always the case. Markets can behave irrationally, leading to significant returns that are not accounted for in a symmetric model.
- Independence of Observations: Many investors treat day-to-day returns as independent events, but this is often misleading. Investors may react to market movements based on previous trends, leading to either positive or negative correlations that disrupt the independence assumption.
These assumptions can cause models to become misaligned with reality. Consequently, investment strategies based on symmetric distributions may fail during volatile market periods. Recognizing this can encourage analysts to adopt more robust models that consider the true nature of asset return behavior.
Real-World Data Challenges
The practicality of applying symmetric distribution is hindered by issues inherent in real-world data. Investors often have to navigate various challenges that make it difficult to apply symmetric models effectively:
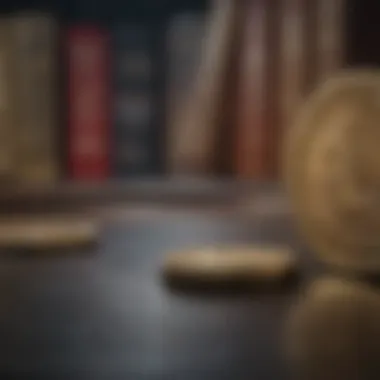
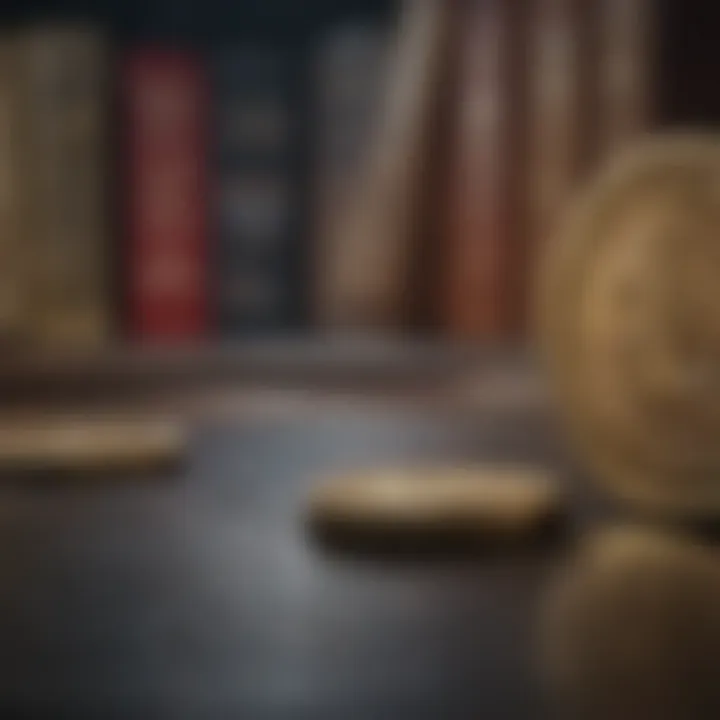
- Inherent Bias: Real-world data can suffer from bias due to a variety of factors, such as timing, selection, and reporting issues. For instance, if certain companies are more frequently studied than others, the resulting data may skew perceptions of performance.
- Outliers: Extreme values can disrupt models based on symmetric distribution. These outliers may not be true reflections of the underlying data set but rather represent unusual market behavior or localized incidents affecting specific stocks. Ignoring these can lead to critical miscalculations.
- Data Availability: Reliable data may not be available for certain investment vehicles, particularly in emerging markets. When data is scarce or unreliable, the foundational premise of using symmetric distributions starts to crumble.
"The reality is that models are only as good as the data behind them. If the data is flawed, the conclusions drawn will reflect that imperfection."
In summary, while symmetric distribution can provide a framework for understanding investment analysis, the limitations derived from assumptions in financial models and challenges with real-world data must be carefully considered. This understanding aids investors in adapting their strategies, ensuring they take into account the more chaotic nature of financial markets and avoid the trap of relying too heavily on simplified distributions.
Case Studies
The examination of case studies plays a pivotal role in understanding the real-world applications of symmetric distribution in investment analysis. These studies add substantial depth to the theoretical frameworks by showcasing actual scenarios where symmetry in data distribution has influenced decision-making and outcomes. They allow investors and financial analysts to glean insights from past behaviors, providing a practical lens through which to view abstract concepts. By examining historical data and behavioral finance perspectives, we can paint a clearer picture of the advantages and constraints of symmetric distributions.
Historical Market Trends
Market Crashes and Corrections
Market crashes and corrections are significant events that provide a fertile ground for analysis through the lens of symmetric distribution. The characteristic that makes these events particularly compelling is their symmetry in terms of price deviations from a mean or trend. While one might think of crashes as extreme anomalies, they often reveal a symmetrical pattern when scrutinized over longer periods.
By analyzing past crashes, one can identify recurring patterns that could be beneficial for predicting future downturns. For instance, historical data shows that many market corrections return to their pre-crash levels over time, suggesting that the distribution of returns can reflect a symmetrical pattern.
However, it's crucial to recognize the unique feature of market crashes: they often skew perceptions of risk. During periods of correction, investors may fall prey to recency bias, leading them to believe that the past is a good predictor of the future. This can create an illusion of symmetry that fails to account for systemic changes or unprecedented events, such as the financial crisis of 2008, where underlying factors fundamentally altered market dynamics. Thus, while these patterns can guide investment strategies, relying solely on historical trends without context can lead to misguided decisions.
Long-Term Growth Patterns
On the flip side, long-term growth patterns also contribute significantly to our understanding of symmetric distribution. This aspect highlights a key characteristic: the general upward trajectory of markets over extended periods, punctuated by corrections. Long-term analysis often reveals that despite short-term volatility, markets tend to move toward a mean growth rate driven by economic fundamentals. This property can be particularly attractive for investors focused on building wealth over time.
A unique feature of long-term growth is its resilience. Unlike short-term fluctuations, which can appear chaotic and volatile, long-term trends exhibit a level of steadiness that can be essential for strategic planning. Investors who focus on these trends can leverage them for informed decision-making, especially when considering portfolio allocation and risk management strategies.
However, one should also be cautious. Long-term growth projections can become overly optimistic, leading to a poor assessment of risk. For instance, the dot-com bubble in the early 2000s demonstrated how assuming that growth would continue without recognizing shifts in technology and consumer behavior could result in significant losses. Therefore, while long-term growth can present a symmetric distribution pattern, it demands critical scrutiny of the underlying factors that support that growth.
Behavioral Finance Perspectives
Behavioral finance provides yet another dimension to our understanding of symmetric distributions in market behavior. This perspective challenges the purely rational models of investment analysis. Investors often behave in ways that exhibit both rationality and emotional responses, leading to outcomes that align with symmetric distributions in some contexts but detract from it in others.
For example, during a market rally, investors may become overly optimistic, leading to a perception that price increases will continue indefinitely. This psychological behavior can lead to price deviations from the mean that appear symmetrical in the short run, but may not hold true as fundamental principles govern long-term valuations. Understanding these behavioral nuances is crucial for investors aiming to navigate the complex interplay between market reality and psychological perceptions.
Culmination
In wrapping up our exploration of symmetric distribution in investment analysis, it’s imperative to underscore its vital role in fostering a nuanced understanding of financial data. Symmetric distributions serve as a foundational concept, enabling investors to grasp the underlying patterns of market behaviors that can deeply influence decision-making processes. The equilibrium reflected in symmetric distributions allows analysts to make predictions based on historical data, ensuring a more informed investment approach.
Summary of Key Findings
Throughout this article, several key findings emerge that solidify the rationale for integrating symmetric distributions into investment strategies:
- Foundation of Financial Models: Symmetric distributions, particularly normal and uniform types, are often assumed in financial models, acting as a springboard for risk assessment methodologies.
- Clarity in Data Interpretation: Investors benefit from the clear understanding provided by the mean, median, and mode being equivalent in symmetric distributions, which simplifies data interpretation and aids in effective financial forecasting.
- The Role of Central Limit Theorem: The Central Limit Theorem's implications highlight how sampling distributions tend towards normality, stressing the reliability of symmetric distribution in analyzing large datasets.
- Practical Application: Real-world applications, especially in portfolio management and risk assessment, are enriched by energy from symmetric distributions. Tools like Efficient Frontier and Mean-Variance Analysis provide refined methods for optimizing investment choices.
Future of Symmetric Distribution in Finance
Looking ahead, the role of symmetric distribution in finance appears promising but requires adaptation in light of contemporary challenges. As markets evolve and exhibit greater volatility and asymmetric behaviors, traditional reliance on symmetric models will need reconsideration. Some potential trajectories include:
- Integration with Asymmetric Models: As market behaviors become less predictable, merging insights from symmetric data with concepts from asymmetric distributions can enhance predictive power. Adjusting traditional methods to acknowledge the realities of fat tails and extreme events is crucial.
- Advanced Analytical Software: The rising sophistication of analytical software will aid investors in visualizing complex data scenarios, allowing for a richer tapestry of insights that includes both symmetric and asymmetric traits.
- Behavioral Finance Insights: Insights from behavioral finance can provide a fresh perspective on investor behavior, leading to a deeper understanding of how perceptions and biases influence market dynamics, thus offering more nuanced applications of symmetric distribution concepts.
In essence, while symmetric distributions have been a stalwart in financial analysis, the future may call for a hybrid approach that embraces the complexities of the real world.
As we navigate through an increasingly intricate financial landscape, recognizing the nuances of symmetric distribution will empower investors and analysts alike to make shrewder, more informed decisions.